Over the two years since generative AI (gen AI) emerged, much of the enterprise focus on the technology has revolved around its ability to foster increased productivity for software engineers and developers. Yet while such efficiency gains are a significant driver of the $2.6 trillion to $4.4 trillion that McKinsey estimates gen AI could add to the global economy, a growing number of organizations are taking a more expansive view of the technology’s full impact on the entire process of creating software products.
By integrating all forms of AI into the end-to-end software product development life cycle (PDLC), companies can empower product managers (PMs), engineers, and their teams to spend more time on higher-value work and less on routine tasks. As part of this broad shift, they can incorporate more robust sources of data and feedback in a new development framework that prioritizes customer-centric solutions. This holistic redesign should ultimately accelerate the process, improve product quality, increase customer adoption and satisfaction, and spur greater innovation (Exhibits 1 and 2). Industry leaders share this perspective. Inbal Shani, chief product officer (CPO) and head of R&D at Twilio, emphasizes the transformative potential of AI: “With the implementation of AI, I believe the most relevant and unique change will be improvements in the quality of products, given the ability to better analyze, synthesize information, and make recommendations.”
Reaching that lofty end goal doesn’t happen overnight. An AI transformation of the software PDLC involves several changes, each bringing its share of necessary adaptation and potential challenges. Based on our research and interviews with industry leaders, this article examines five individual, critical shifts that AI is poised to bring to the software PDLC, as well as some key implications and impacts this broad redesign will have on various aspects of product organizations, including business model, capabilities, organizational structure, and data governance.
AI’s five critical shifts for the software PDLC
As part of its broad overhaul of the product development process, AI is poised to bring five fundamental changes to the software PDLC, which should profoundly impact speed, quality, value, and overall innovation.
1. Significantly faster time to market
Many organizations are already experiencing the time-saving impact of gen AI in software engineering, but gen AI can do the same for the entire product management and development process. Much of this acceleration comes by shortening the journey from the early strategy and vision stages to late-stage product deployment and scaling. AI’s ability to automate time-consuming routine tasks such as project management, market analysis, performance testing, and feedback analysis and documentation frees PMs, engineers, and designers to focus on higher-value, more satisfying tasks requiring human creativity and judgment. These range from product vision and strategy setting to concept development and feature prioritization.
This shift not only speeds up the development cycle but also offers unprecedented opportunities for innovation. The time savings and information AI tools provide can allow teams to create multiple iterations of a product, improving its market fit. As part of this acceleration, organizations should be able to conduct faster market testing and respond more swiftly to user feedback, consumer changes, and shifting dynamics or trends, which should result in better products.
2. Products deliver customer value much sooner
Successful product development depends on realizing the value of market-relevant ideas. While development teams usually start with the right intent, it has typically taken a few releases to listen to and incorporate customer feedback to deliver real value. AI is remaking this process by integrating fragmented data sources of customer feedback and product usage into the development cycle, making it possible to build products in an accelerated manner that are linked to customer value from the outset.
For example, AI can stitch together data from initial customer research during the discovery and viability phases along with telemetry, service ticket data, and support feedback to track impact end to end. AI tools can also integrate real-time customer input, social media sentiment/ interactions, and competitive research alongside historical data and market trends. Teams using this approach can gain actionable insights to help create more rigorous and customer-centric product concepts, thus increasing successful launches. Stack Overflow, an online developer community, uses AI to efficiently comb through past and current customer research and feedback and provide insights as teams iterate. “We are entering a new economy where knowledge as a service will power the future,” says Prashanth Chandrasekar, the company’s CEO. “This new set of business models depends on a community of creators that progressively create new and relevant domain-specific content based on high-quality, validated, and trustworthy data. Our product is always being updated to reflect what customers want and leveraging the powerful synergies between Stack Overflow and its AI partners, bringing a new level of productivity to the developer ecosystem through cutting-edge tools backed by an accurate data foundation trusted by millions of developers.”
Would you like to learn more about our Technology, Media & Telecommunications Practice?
3. More good ideas see the light of day
Some of the most essential but time-consuming and costly parts of the traditional software PDLC are identifying (or discovering) market opportunities and testing the viability of ideas for solving problems in a way that will appeal to customers. These two phases have typically had to be separate processes. The traditional prototyping process required so much time and resources—and involved so much risk—that companies had to focus initially on planning and research. AI eliminates that strict dividing line, enabling quick prototyping and automated A/B testing to validate various hypotheses and identify promising ideas. This lays the foundation for rapid iteration and data-driven decision making.
Using AI for prototyping and testing new product ideas significantly reduces the resources required in the viability stage. As a result, product teams can run many more experiments, raising the odds of promising ideas getting due consideration. Organizations can also use AI to eliminate some of the guesswork. For example, organizations no longer have to take the sometimes unreliable step of stack-ranking ideas before testing their viability. Without data informing this decision-making process, the process has always been susceptible to the undue influence of the loudest voice in the room or the highest-paid person’s opinion, known as HiPPO bias. While it seems unrealistic to think that AI will do away with hierarchy-driven decisions entirely, it does have the potential to reduce the subjectivity of the process.
Twilio’s Shani thinks the impact of AI’s perceived impartiality could be significant. “Having more data points can change business leaders’ strategy and prioritization decisions,” she says. “AI can help analyze data sets and be an unbiased element in the conversation. After strategic decisions are made, AI can then help continuously monitor metrics and evaluate the progress.” In this way, AI accelerates the development cycle and increases the odds that resources will be allocated to the most promising ideas, reducing costs and the chances of failure.
Reddit’s product team exemplifies this approach. Its engineers use AI to help define and prototype innovative features rapidly. “New feature definition, prototyping, and testing are all happening in parallel and faster than ever before,” notes Pali Bhat, the company’s CPO. “Our teams can now dream up an idea one day and have a functional prototype the next. It’s that fast.”
4. The idea of PMs as “mini-CEOs” finally comes to fruition
The traditional software PDLC has been a less than seamless undertaking. PMs have had to rely on a team of specialized roles to fulfill many essential tasks, with several handoffs taking place before launch. With AI, PMs can “shift right” (in industry parlance), taking on additional duties and oversight in the later stages of the process. They will now have the ability and capacity to have end-to-end oversight from ideation to value realization. They will run discovery and leverage AI tools to rapidly prototype products, create marketing collateral (including product one-pagers and pitch decks), and build technical proofs-of-concept (POCs) with minimal involvement of product marketers, designers, and engineers. PMs can also take on more complex and valuable work, such as long-term strategy planning, creative problem solving, interviewing key customers and stakeholders, and assessing use cases.
This expansion of capabilities should allow PMs to move ideas from conception to tangible demonstrations more rapidly, with more end-to-end accountability, blurring the lines between product management, product design, and product marketing. In the process, this can also create better PMs. Just as gen AI tools can synthesize customer feedback, they can analyze meeting notes and call transcripts to provide valuable internal feedback, helping employees learn and receive coaching more frequently and efficiently.
As part of this expansion of responsibilities, the PM role may well subsume other separate positions like product marketing manager (PMM), product owner (PO), technical product manager (TPM), and user interface (UI) and user experience (UX) roles. Eventually, with AI tools enhancing PM capabilities in areas traditionally owned by other roles—such as market analysis and messaging or customer-centric research—the gradual convergence of positions may make the most sense. “The PMM and PM role will most likely converge under the same product organization,” says Varun Parmar, general manager at Adobe and former CPO and COO of Miro. “As more PMM tasks such as messaging become automated by AI, the PMM function will need to go really deep into positioning and become fully integrated within the product team.”
5. Quality, risk, compliance, and accessibility are addressed in parallel with coding and building
Given how much AI is expected to accelerate the entire software PDLC, organizations will want to embed risk, compliance, and accessibility testing earlier to avoid the risk of overlooking potentially costly errors or defects. Instead of being addressed at a relatively late stage, the issues will be top of mind for decision-making teams starting in discovery. This should help ensure product ideas align with requirements, while laying the foundation for such safeguards to be an ongoing part of the software PDLC. Product teams, for instance, will need to include assessments and automatically apply coding standards and requirements throughout the development process.
AI makes this “shift left” (in tech vernacular) in the process necessary and can also help enable it. Solutions range from automated code compliance and accessibility checks to threat detection and prevention to vulnerability scanning when developers write code. For example, GitHub recently announced functionality that allows companies to set enterprise-wide security and compliance guidelines across code repositories so that developers’ code can be compliant immediately. Additionally, GitHub Copilot is speeding up code reviews by up to seven times, finding and fixing vulnerabilities and stylistic issues.
“The greatest value will occur when we can remove the bottlenecks and ensure our products are compliant and high-quality from the get-go,” Reddit’s Bhat notes. “We are already doing that with accessibility, where we ensure that it is ‘built in’ from the start of the PDLC rather than bolting it on after the fact.” By analyzing test results, teams can identify patterns and predict potential problem areas, enabling continuous quality enhancements. This early-stage testing for risk, compliance, and accessibility should ultimately enable higher-quality products that experience lower churn.
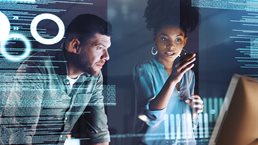
Navigating the generative AI disruption in software
What this means for organizations
The shifts AI is poised to bring to the software PDLC will have profound implications for how product organizations are structured and operate. While some effects are already becoming apparent, others are less certain as leaders grapple with complex questions about how this new era will evolve.
Business model
As AI brings together disparate data sources, and software companies can link products to customer value much sooner than in the past, customers will increasingly expect a business model in which they pay based on outcomes rather than usage. Until recently, that has practically been unfeasible, with the fragmentation of accountability and data within the traditional software PDLC hindering companies from reliably measuring and clearly demonstrating the value realized from a given product. The data platform connectivity and the growth in the PM’s role and responsibilities ushered in by AI should enable companies to link pricing to end-to-end value delivery. Some pioneering organizations are already experimenting with outcome-based pricing for select offerings—for example, marketing lead generation software. While it is still early days, with potential challenges surrounding visibility and control of end-to-end outcomes on the horizon, such a model is likely to become increasingly prevalent as more customers demand that providers make commitments that their products will deliver specific results.
Tooling and platforms
To embed AI into the software PDLC, organizations will need to invest in new AI tools beyond coding assistance, including a comprehensive data platform and tools for AI-enhanced product development. However, relying on too many narrowly targeted tools can create a fragmented and suboptimal process. Instead, organizations may want to work toward integrated solutions that govern the entire developer stack. “The proliferation of point solutions is fragmenting the developer experience,” Reddit’s Bhat argues. “Engineering teams can only effectively use a certain number of tools as part of their core workflow. Integration will become more crucial, and we will see toolchain consolidation over time.”
Bringing various development tools together is just the beginning. Industry leaders are considering whether separate product management, design, and development tools will eventually become one integrated solution. Such a fully integrated model would minimize the possibility of complicated handoffs or error-prone transitions along the journey from product road map to designer mockups to working code, enabling real-time co-creation and more informed decision making. Twilio’s Shani says, “Companies should invest more in tools that enhance productivity across roles, connecting the dots between discovery and commercialization.”
One of the biggest challenges in the standard software PDLC that Shani highlights is the lack of data connectivity. A robust, AI-enhanced integrated data platform will be crucial for informed product decisions, consolidating diverse data sources from development artifacts to user feedback and market intelligence. An AI assistant analyzing this data can trace a feature’s journey, identify correlations, and predict issues based on historical patterns. Only by breaking down data silos in this way can companies gain a holistic view of their product life cycle, enabling more user-centric decision making.
Talent and organizational structure
The integration of AI into the software PDLC necessitates some significant changes in organizational skill sets. As AI accelerates the timeline and automates certain low- and midlevel tasks, companies may need to rethink their talent mix and apprenticeship model to take advantage of these shifts. With gen AI automating more basic coding, for instance, there may be an increased need for senior/staff (L2/L3) engineers who can expertly navigate complex architecture and review AI-generated code. Thomas Dohmke, CEO of GitHub, stresses that “knowing how to figure out whether the content provided by the AI is actually the right answer is going to be crucial.”
The demand for more advanced skill sets will shift the labor pyramid toward senior engineers, yet how this will impact the mentoring and nurturing of new generations of engineers is still to be determined. After all, maintaining a robust pipeline of senior engineers requires strong onboarding and structured capability building, including giving junior talent early responsibility and providing frequent feedback. One answer may be that people do more of it on their own. Thanks largely to open-source contributions through platforms like GitHub, the paths for learning how to build mission-critical software are expanding and democratizing such that aspiring engineers can now effectively undertake self-apprenticeships.
Other important AI-enabled shifts for product-related technical capabilities include the following:
-
Demand for skills solely focused on UI design will decrease, while the criticality of UX researchers skilled in “human-in-the-loop” design will rise. Microsoft’s Ritcha Ranjan, VP Office AI, predicts, “On the design side, AI is enabling teams to prototype faster than ever before. However, this shift highlights a growing need for human expertise in spatial reasoning and systems thinking—skills that are essential for creating coherent and delightful products.” This is particularly useful to navigate the challenges that adopting these tools will bring, such as bias in AI models and data sets, or user frustration due to the “loss of human touch,” as Ranjan puts it.
Site reliability engineering (SRE) tasks are already becoming automated, with AI taking over system checks, log analysis, and incident triage. This trend should continue under an AI software PDLC, enabling SRE roles to concentrate on higher-value work, such as developing tools for proactive maintenance, predictive anomaly detection, and self-healing systems, enhancing overall system reliability and performance.
- AI will push developers toward full-stack proficiency and require them to become AI-stack developers. With AI tools increasingly handling UI coding and automating routine operations, front-end developers will be expected to gradually transition into full-stack developer roles driving end-to-end innovation. Furthermore, this full-stack talent will be required to understand the tech and business implications of integrating AI into what they are building, such as the cost of AI capabilities. “AI is changing the product development life cycle by shifting human effort toward areas where we add the most value—those requiring deeper reasoning and problem solving,” notes Microsoft’s Ranjan. “Traditionally, engineers follow a process: scoping requirements, determining system integration, shaping the solution, writing code, testing, reviewing with peers, and refining the design. Today, AI accelerates code writing and testing. As the technology evolves, it will begin tackling more complex aspects of the process, but advancements will be limited to textural chains of thought to emulate human reasoning. These advancements will continue to shift where engineers focus their time.”
- The new PDLC may redefine (or obviate) software development engineer in test (SDET) roles. As AI-enabled tools increasingly handle unit testing, integration testing, and predictive anomaly detection, organizations might consider absorbing testing into broader development responsibilities, including reviewing AI-generated code, devising testing strategies, and ensuring overall software quality.
Beginning the AI transformation of the software PDLC
To fully leverage the AI-enabled software PDLC, companies will likely need to transform their approach across multiple dimensions. Organizationally, this involves investing in AI talent and upskilling existing employees, particularly in R&D, to meet shifting labor demands. Strategically, companies should prioritize diverse data sources to inform product decisions and realign business planning around outcome-based, data-driven metrics that fuel a focus on user adoption and continuous product evolution. Operationally, investing in AI-enhanced tools and integrated platforms can streamline development, foster cross-functional collaboration, and accelerate time to market.
However, organizations may only realize the benefits of an AI-enabled software PDLC with a fundamental shift in their ways of working. Just as agile tools didn’t enable PMs to create business value faster, and DevOps tools didn’t allow engineers to release more frequent updates without adopting new roles and operating models, merely adopting AI tools isn’t enough to transform the software PDLC. Companies will want to strongly consider investing in new ways of working—retraining their teams to align with new organizational structures, talent and capabilities, and tooling and platform shifts.
Ultimately, this holistic transformation should result in higher-quality products reaching customers faster, putting their needs at the center of the development process and delivering greater value (to them and software providers) in the AI-enabled future.