Artificial intelligence has arrived in the workplace and has the potential to be as transformative as the steam engine was to the 19th-century Industrial Revolution.1 With powerful and capable large language models (LLMs) developed by Anthropic, Cohere, Google, Meta, Mistral, OpenAI, and others, we have entered a new information technology era. McKinsey research sizes the long-term AI opportunity at $4.4 trillion in added productivity growth potential from corporate use cases.2
Therein lies the challenge: the long-term potential of AI is great, but the short-term returns are unclear. Over the next three years, 92 percent of companies plan to increase their AI investments. But while nearly all companies are investing in AI, only 1 percent of leaders call their companies “mature” on the deployment spectrum, meaning that AI is fully integrated into workflows and drives substantial business outcomes. The big question is how business leaders can deploy capital and steer their organizations closer to AI maturity.
This research report, prompted by Reid Hoffman’s book Superagency: What Could Possibly Go Right with Our AI Future,3 asks a similar question: How can companies harness AI to amplify human agency and unlock new levels of creativity and productivity in the workplace? AI could drive enormous positive and disruptive change. This transformation will take some time, but leaders must not be dissuaded. Instead, they must advance boldly today to avoid becoming uncompetitive tomorrow. The history of major economic and technological shifts shows that such moments can define the rise and fall of companies. Over 40 years ago, the internet was born. Since then, companies including Alphabet, Amazon, Apple, Meta, and Microsoft have attained trillion-dollar market capitalizations. Even more profoundly, the internet changed the anatomy of work and access to information. AI now is like the internet many years ago: The risk for business leaders is not thinking too big, but rather too small.
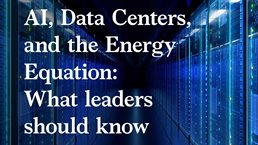
Register for upcoming McKinsey Live
Amid the AI boom, compute power is emerging as one of this decade's most critical resources, influencing the pace at which AI is deployed. Data centers train the foundation models and machine learning applications that underpin all AI technology. The hardware, processors, memory, storage, and energy needed to operate these data centers are collectively known as compute power—and there is a seemingly unquenchable need for more.
Meeting this demand is not simply a race to invest trillions of dollars. Join McKinsey senior partners Humayun Tai and Pankaj Sachdeva on April 23 at 10:30a.m. EDT / 4:30p.m. CEST as they share how business leaders can navigate this complex environment.
This report explores companies’ technology and business readiness for AI adoption (see sidebar “About the survey”). It concludes that employees are ready for AI. The biggest barrier to success is leadership.
Chapter 1 looks at the rapid advancement of technology over the past two years and its implications for business adoption of AI.
Chapter 2 delves into the attitudes and perceptions of employees and leaders. Our research shows that employees are more ready for AI than their leaders imagine. In fact, they are already using AI on a regular basis; are three times more likely than leaders realize to believe that AI will replace 30 percent of their work in the next year; and are eager to gain AI skills. Still, AI optimists are only a slight majority in the workplace; a large minority (41 percent) are more apprehensive and will need additional support. This is where millennials, who are the most familiar with AI and are often in managerial roles, can be strong advocates for change.
Chapter 3 looks at the need for speed and safety in AI deployment. While leaders and employees want to move faster, trust and safety are top concerns. About half of employees worry about AI inaccuracy and cybersecurity risks. That said, employees express greater confidence that their own companies, versus other organizations, will get AI right. The onus is on business leaders to prove them right, by making bold and responsible decisions.
Chapter 4 examines how companies risk losing ground in the AI race if leaders do not set bold goals. As the hype around AI subsides, companies should put a heightened focus on practical applications that empower employees in their daily jobs. These applications can create competitive moats and generate measurable ROI. Across industries, functions, and geographies, companies that invest strategically can go beyond using AI to drive incremental value and instead create transformative change.
Chapter 5 looks at what is required for leaders to set their teams up for success with AI. The challenge of AI in the workplace is not a technology challenge. It is a business challenge that calls upon leaders to align teams, address AI headwinds, and rewire their companies for change.
Imagine a world where machines not only perform physical labor but also think, learn, and make autonomous decisions. This world includes humans in the loop, bringing people and machines together in a state of superagency that increases personal productivity and creativity (see sidebar “AI superagency”). This is the transformative potential of AI, a technology with a potential impact poised to surpass even the biggest innovations of the past, from the printing press to the automobile. AI does not just automate tasks but goes further by automating cognitive functions. Unlike any invention before, AI-powered software can adapt, plan, guide—and even make—decisions. That’s why AI can be a catalyst for unprecedented economic growth and societal change in virtually every aspect of life. It will reshape our interaction with technology and with one another.
Scientific discoveries and technological innovations are stones in the cathedral of human progress.
Many breakthrough technologies, including the internet, smartphones, and cloud computing, have transformed the way we live and work. AI stands out from these inventions because it offers more than access to information. It can summarize, code, reason, engage in a dialogue, and make choices. AI can lower skill barriers, helping more people acquire proficiency in more fields, in any language and at any time. AI holds the potential to shift the way people access and use knowledge. The result will be more efficient and effective problem solving, enabling innovation that benefits everyone.
Over the past two years, AI has advanced in leaps and bounds, and enterprise-level adoption has accelerated due to lower costs and greater access to capabilities. Many notable AI innovations have emerged (Exhibit 1). For example, we have seen a rapid expansion of context windows, or the short-term memory of LLMs. The larger a context window, the more information an LLM can process at once. To illustrate, Google’s Gemini 1.5 could process one million tokens in February 2024, while its Gemini 1.5 Pro could process two million tokens by June of that same year.4 Overall, we see five big innovations for business that are driving the next wave of impact: enhanced intelligence and reasoning capabilities, agentic AI, multimodality, improved hardware innovation and computational power, and increased transparency.
Image description begins:
The text-based exhibit illustrates the evolution of capabilities of several gen AI large language models, or LLMs, from select frontier labs between 2022 and 2025. The is presented as a table comparing two time periods: 2022-2023 and January 2025. For each of five LLMs—Anthropic's Claude, Google's Gemini, Meta's Llama, Microsoft's Phi, and OpenAI's GPT—the exhibit shows a list of capabilities for each time period. In 2022-2023, all five platforms lacked multimodal capabilities, functioning primarily with text only. Anthropic's Claude, for example, showed limited contextual understanding and no tool usage. Google's Gemini, similarly, had limited real-time data integration and low personalization. Meta's Llama 1 exhibited fair reasoning but had difficulty with complex conversations and lacked API access. Microsoft's Phi-1 had fair reasoning limited to coding tasks, with focused training on a smaller dataset. OpenAI's GPT-3.5 demonstrated fair reasoning, scoring well on the SAT but poorly on the bar examination, while also displaying limited contextual understanding in complex conversations, though it did offer standard API access for text generation.
By January 2025, a significant shift is apparent. Claude 3.5, Gemini 2.0 Flash, Llama 3.3, Phi-4, and OpenAI's model o1 all gained multimodal capabilities, incorporating text, audio, and images. Advanced reasoning capabilities, capable of multistep problem-solving and nuanced analysis, became common across most of the platforms. Enhanced contextual understanding, maintaining coherence during long dialogues, is also highlighted as an improvement. Furthermore, real-time data integration and advanced personalization features were added to some platforms. Finally, several platforms highlight improved or advanced API access, allowing for tools related to model and agent development and multimodal inputs. Source: Company websites and press releases. This image description was completed with the assistance of Writer, a gen AI tool.
Image description ends.
Intelligence and reasoning are improving
AI is becoming far more intelligent. One indicator is the performance of LLMs on standardized tests. OpenAI’s Chat GPT-3.5, introduced in 2022, demonstrated strong performance on high-school-level exams (for example, scoring in the 70th percentile on the SAT math and the 87th percentile on the SAT verbal sections). However, it often struggled with broader reasoning. Today’s models are near the intelligence level of people who hold advanced degrees. GPT-4 can so easily pass the Uniform Bar Examination that it would rank in the top 10 percent of test takers,5 and it can answer 90 percent of questions correctly on the US Medical Licensing Examination.6
The advent of reasoning capabilities represents the next big leap forward for AI. Reasoning enhances AI’s capacity for complex decision making, allowing models to move beyond basic comprehension to nuanced understanding and the ability to create step-by-step plans to achieve goals. For businesses, this means they can fine-tune reasoning models and integrate them with domain-specific knowledge to deliver actionable insights with greater accuracy. Models such as OpenAI’s o1 or Google’s Gemini 2.0 Flash Thinking Mode are capable of reasoning in their responses, which gives users a human-like thought partner for their interactions, not just an information retrieval and synthesis engine.7
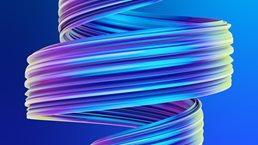
AI in Action: An interactive learning journey
Agentic AI is acting autonomously
I’ve always thought of AI as the most profound technology humanity is working on . . . more profound than fire or electricity or anything that we’ve done in the past.
The ability to reason is growing more and more, allowing models to autonomously take actions and complete complex tasks across workflows. This is a profound step forward. As an example, in 2023, an AI bot could support call center representatives by synthesizing and summarizing large volumes of data—including voice messages, text, and technical specifications—to suggest responses to customer queries. In 2025, an AI agent can converse with a customer and plan the actions it will take afterward—for example, processing a payment, checking for fraud, and completing a shipping action.
Software companies are embedding agentic AI capabilities into their core products. For example, Salesforce’s Agentforce is a new layer on its existing platform that enables users to easily build and deploy autonomous AI agents to handle complex tasks across workflows, such as simulating product launches and orchestrating marketing campaigns.8 Marc Benioff, Salesforce cofounder, chair, and CEO, describes this as providing a “digital workforce” where humans and automated agents work together to achieve customer outcomes.9
Multimodality is bringing together text, audio, and video
Today’s AI models are evolving toward more advanced and diverse data processing capabilities across text, audio, and video. Over the last two years, we have seen improvements in the quality of each modality. For example, Google’s Gemini Live has improved audio quality and latency and can now deliver a human-like conversation with emotional nuance and expressiveness.10 Also, demonstrations of Sora by OpenAI show its ability to translate text to video.11
Hardware innovation is enhancing performance
Hardware innovation and the resulting increase in compute power continue to enhance AI performance. Specialized chips allow faster, larger, and more versatile models. Enterprises can now adopt AI solutions that require high processing power, enabling real-time applications and opportunities for scalability. For example, an e-commerce company could significantly improve customer service by implementing AI-driven chatbots that leverage advanced graphics processing units (GPUs) and tensor processing units (TPUs). Using distributed cloud computing, the company could ensure optimal performance during peak traffic periods. Integrating edge hardware, the company could deploy models that analyze photos of damaged products to more accurately process insurance claims.
Transparency is increasing
AI, like most transformative technologies, grows gradually, then arrives suddenly.
AI is gradually becoming less risky, but it still lacks greater transparency and explainability. Both are critical for improving AI safety and reducing the potential for bias, which are imperative for widescale enterprise deployment. There is still a long way to go, but new models and iterations are rapidly improving. Stanford University’s Center for Research on Foundation Models (CRFM) reports significant advances in model performance. Its Transparency Index, which uses a scale of 1 to 100, shows that Anthropic’s transparency score increased by 15 points to 51 and Amazon’s more than tripled to 41 between October 2023 and May 2024.12
Beyond LLMs, other forms of AI and machine learning (ML) are improving explainability, allowing the outputs of models that support consequential decisions (for example, credit risk assessment) to be traced back to the data that informed them. In this way, critical systems can be tested and monitored on a near-constant basis for bias and other everyday harms that arise from model drift and shifting data inputs, which happens even in systems that were well calibrated before deployment.
All of this is crucial for detecting errors and ensuring compliance with regulations and company policies. Companies have improved explainability practices and built necessary checks and balances, but they must be prepared to evolve continuously to keep up with growing model capabilities.
Achieving AI superagency in the workplace is not simply about mastering technology. It is every bit as much about supporting people, creating processes, and managing governance. The next chapters explore the nontechnological factors that will help shape the deployment of AI in the workplace.
Employees will be the ones to make their organizations AI powerhouses. They are more ready to embrace AI in the workplace than business leaders imagine. They are more familiar with AI tools, they want more support and training, and they are more likely to believe AI will replace at least a third of their work in the near future. Now it’s imperative that leaders step up. They have more permission space than they realize, so it’s on them to be bold and capture the value of AI. Now.
People are using [AI] to create amazing things. If we could see what each of us can do 10 or 20 years in the future, it would astonish us today.
Beyond the tipping point
In our survey, nearly all employees (94 percent) and C-suite leaders (99 percent) report having some level of familiarity with gen AI tools. Nevertheless, business leaders underestimate how extensively their employees are using gen AI. C-suite leaders estimate that only 4 percent of employees use gen AI for at least 30 percent of their daily work, when in fact that percentage is three times greater, as self-reported by employees (Exhibit 2). And while only a total of 20 percent of leaders believe employees will use gen AI for more than 30 percent of their daily tasks within a year, employees are twice as likely (47 percent) to believe they will (see sidebar “Who is using AI at work? Nearly everyone, even skeptical employees”).
The good news is that our survey suggests three ways companies can accelerate AI adoption and move toward AI maturity.
Image description begins:
The exhibit shows the anticipated timeline for US employees' and business leaders’ use of gen AI for more than 30 percent of their daily work tasks, presented as two stacked bar charts, one for C-suite respondents and one for employees. The segments are broken down into five categories representing different timeframes: Already using, less than a year, 1-5 years, over 5 years, and don't anticipate it. A final category, not sure, is also included. A key finding highlighted in the chart is that employees are three times more likely to be using gen AI today than their leaders expect (4 percent of C-suite respondents estimate that employees are currently using gen AI for more than 30 percent of their daily tasks, while 13 percent of employees self-report they are currently doing so). For the C-suite, 16 percent expect employees to start using gen AI for more than 30 percent of their daily tasks within less than a year, 56 percent anticipate such adoption within 1-5 years, 11 percent expect it in over 5 years, and 10 percent don't anticipate employees will ever use gen AI for 30 percent of their work tasks. 3 percent of C-suite respondents are unsure. 34 percent of employees expect to use gen AI for more than 30 percent of their work tasks in less than a year, 37 percent within 1-5 years, 5 percent in over 5 years, and 7 percent don't anticipate ever using it in this way. 4 percent of employees are unsure. Source: McKinsey US CxO survey, Oct–Nov 2024; McKinsey US employee survey, Oct–Nov 2024. This image description was completed with the assistance of Writer, a gen AI tool.
Image description ends.
Leaders can invest more in their employees
As noted at the beginning of this chapter, employees anticipate AI will have a dramatic impact on their work. Now they would like their companies to invest in the training that will help them succeed. Nearly half of employees in our survey say they want more formal training and believe it is the best way to boost AI adoption. They also would like access to AI tools in the form of betas or pilots, and they indicate that incentives such as financial rewards and recognition can improve uptake.
Yet employees are not getting the training and support they need. More than a fifth report that they have received minimal to no support (Exhibit 3). Outside the United States, employees also want more training (see sidebar “Global perspectives on training”).
Image description begins:
The first section of the exhibit is a horizontal bar chart showing the percentage of US employees who believe that specific company initiatives would increase their daily use of gen AI tools. Formal gen AI training from their organization scored highest at 48 percent, followed by seamless integration into existing workflows (45 percent), access to gen AI tools (41 percent), and incentives and rewards (40 percent). Lower percentages were observed for usage of gen AI being a requirement for a certification program (30 percent), explicit instructions from managers to use gen AI (30 percent), being involved in the development of the tools (29 percent), and OKRs/KPIs tied to gen AI usage (22 percent).
The second section is a stacked pair of segmented bar charts illustrating the perceived level of support for gen AI capability building at their organizations, comparing current vs in three years. This chart shows the distribution of responses across four levels of support: not needed, none/minimal, moderate to significant, and fully supported. Currently, 6 percent of employees report that support for gen AI in their organizations is not needed, 22 percent report they receive none/minimal support, 44 percent report moderate to significant support, and 29 percent report they are fully supported. Looking ahead to three years in the future, these percentages are projected to shift considerably: gen AI support not needed drops to 4 percent, none/minimal support for gen AI usage decreases to 10 percent, moderate to significant support for gen AI usage increases to 56 percent, and fully supported increases to 31 percent. Source: McKinsey US employee survey, Oct–Nov 2024. This image description was completed with the assistance of Writer, a gen AI tool.
Image description ends.
Global perspectives on training
To get a clearer picture of global AI adoption trends, we looked at trends across five countries: Australia, India, New Zealand, Singapore, and the United Kingdom. Broadly speaking, these employees and C-suite leaders—the “international” group in this report—have similar views of AI as their US peers. In some key areas, however, including the topic of training, their experiences differ.
Many international employees are concerned about insufficient training, even though they report receiving far more support than US employees. Some 84 percent of international employees say they receive significant or full organizational support to learn AI skills, versus just over half of US employees. International employees also have more opportunities to participate in developing gen AI tools at work than their US counterparts, with differences of at least ten percentage points in activities such as providing feedback, beta testing, and requesting specific features (exhibit).
C-suite leaders can help millennials lead the way
Many millennials aged 35 to 44 are managers and team leaders in their companies. In our survey, they self-report having the most experience and enthusiasm about AI, making them natural champions of transformational change. Millennials are the most active generation of AI users. Some 62 percent of 35- to 44-year-old employees report high levels of expertise with AI, compared with 50 percent of 18- to 24-year-old Gen Zers and 22 percent of baby boomers over 65 (Exhibit 4). By tapping into that enthusiasm and expertise, leaders can help millennials play a crucial role in AI adoption.
Image description begins:
The exhibit is a grid of proportional area charts displaying US employee sentiment toward gen AI by age group. Each row represents a different sentiment, from top to bottom: has extensive familiarity with gen AI, is comfortable using gen AI at work, provides feedback on gen AI tools, and wants to participate in the design of gen AI tools. The columns represent age groups: 18-24, 25-34, 35-44, 45-54, 55-64, and 65+. The data is presented as percentages of respondents who agreed with each sentiment within each age group.
The chart reveals that the 35-44 age group exhibits the most positive sentiment across most categories. For example, 90 percent of this group reports being comfortable using gen AI at work, the highest percentage among all age groups for this metric. This group also shows the highest percentage (62 percent) reporting extensive familiarity with gen AI. In contrast, the 55-64 and 65+ age groups consistently show lower percentages across all four metrics, with only 26 percent and 22 percent of employees in these age groups reporting extensive familiarity with gen AI respectively. The 18-24, 25-34, and 45-54 age groups show intermediate levels of positive sentiment, generally lower than the 35-44 group but higher than the 55-64 and 65+ age groups. Source: McKinsey US employee survey, Oct–Nov 2024. This image description was completed with the assistance of Writer, a gen AI tool.
Image description ends.
Since many millennials are managers, they can support their teams to become more adept AI users. This helps push their companies toward AI maturity. Two-thirds of managers say they field questions from their team about how to use AI tools at least once a week, and a similar percentage say they recommend AI tools to their teams to solve problems (Exhibit 5).
Image description begins:
The exhibit examines US manager respondents and their experiences with gen AI tools. The exhibit is composed of two main sections.
The top section of the exhibit examines the frequency of inquiries that managers field from their employees about using new gen AI tools at work. This is depicted as a horizontal bar chart showing percentages of respondents. 5 percent of managers report less than quarterly inquiries; 5 percent report quarterly inquiries; 12 percent report inquiries once a month; 15 percent report once a week; 28 percent report a few times a week; 9 percent report once a day; and 16 percent report multiple times a day. Finally, 10 percent of report not at all.
The second section explores the use of gen AI tools to resolve team member challenges. This section uses two donut charts, each showing percentages of respondents. The first donut chart indicates that 68 percent of managers report recommending a gen AI tool to solve a team member's challenge in the past month. The second donut chart shows that 86 percent of managers who recommended a gen AI tool report that the tool was successful in resolving the team member’s challenge. Source: McKinsey US employee survey, Oct–Nov 2024. This image description was completed with the assistance of Writer, a gen AI tool.
Image description ends.
Since leaders have the permission space, they can be bolder
In many transformations, employees are not ready for change, but AI is different. Employee readiness and familiarity are high, which gives business leaders the permission space to act. Leaders can listen to employees describe how they are using AI today and how they envision their work being transformed. They also can provide employees with much-needed training and empower managers to move AI use cases from pilot to scale.
It’s critical that leaders meet this moment. It’s the only way to accelerate the probability that their companies will reach AI maturity. But they must move with alacrity, or they will fall behind.
AI technology is advancing at record speed. ChatGPT was released about two years ago; OpenAI reports that usage now exceeds 300 million weekly users13 and that over 90 percent of Fortune 500 companies employ its technology.14 The internet did not reach this level of usage until the early 2000s, nearly a decade after its inception.
Soon after the first automobiles were on the road, there was the first car crash. But we didn’t ban cars—we adopted speed limits, safety standards, licensing requirements, drunk-driving laws, and other rules of the road.
The majority of employees describe themselves as AI optimists; Zoomers and Bloomers make up 59 percent of the workplace. Even Gloomers, who are one of the two less-optimistic segments in our analysis, report high levels of gen AI familiarity, with over a quarter saying they plan to use AI more next year.
Business leaders need to embrace this speed and optimism to ensure that their companies don’t get left behind. Yet despite all the excitement and early experimentation, 47 percent of C-suite leaders say their organizations are developing and releasing gen AI tools too slowly, citing talent skill gaps as a key reason for the delay (Exhibit 6).
Image description begins:
The exhibit shows US C-suite executive sentiment toward the pace of development and release of gen AI tools within their organizations, in the form of two segmented bar charts.
The first bar chart presents the overall perception of the pace, where 47 percent of respondents find the pace to be too slow, while 45 percent feel it is about right, and a smaller 9 percent consider it too fast. The second bar chart delves into the top reasons behind the perceived slow pace of gen AI tool development and release in executives’ organizations, focusing on the responses from those who indicated that development was too slow. The most prominent reason cited is talent skill gaps, accounting for 46 percent of these responses. Resourcing constraints followed closely, with 38 percent of respondents identifying this as a key factor. Complex approval process and technical complexity each receive 8 percent of the responses. Source: McKinsey US CxO survey, Oct-Nov 2024. This image description was completed with the assistance of Writer, a gen AI tool.
Image description ends.
Business leaders are trying to meet the need for speed by increasing investments in AI. Of the executives surveyed, 92 percent say they expect to boost spending on AI in the next three years, with 55 percent expecting investments to increase by at least 10 percent from current levels. But they can no longer just spend on AI without expecting results. As companies move on from the initial thrill of gen AI, business leaders face increasing pressure to generate ROI from their gen AI deployments.
We are at a turning point. The initial AI excitement may be waning, but the technology is accelerating. Bold and purposeful strategies are needed to set the stage for future success. Leaders are taking the first step: One quarter of those executives we surveyed have defined a gen AI road map, while just over half have a draft that is being refined (Exhibit 7). With technology changing this fast, all road maps and plans will evolve constantly. For leaders, the key is to make some clear choices about what valuable opportunities they choose to pursue first—and how they will work together with peers, teams, and partners to deliver that value.
Image description begins:
The exhibit is comprised of two horizontal segmented bar charts. The first chart displays the share of US C-suite respondents who have a defined gen AI roadmap. 21 percent report not currently having a roadmap but one was in progress, 53 percent indicate having a roadmap that is still being refined, and 25 percent state that a comprehensive roadmap is already in place.
The second bar chart illustrates the level to which US C-suite respondents have identified revenue-generating use cases for gen AI. 1 percent of respondents indicate they have not yet identified any such use cases, while 10 percent report they have minimally identified, 38 percent have partially identified, 39 percent have mostly identified, and 12 percent have fully identified such use cases. Source: McKinsey US CxO survey, Oct-Nov 2024. This image description was completed with the assistance of Writer, a gen AI tool.
Image description ends
The dilemma of speed versus safety
There’s a spanner in the works: Regulation and safety often continue to be seen as insurmountable challenges rather than opportunities. Leaders want to increase AI investments and accelerate development, but they wrestle with how to make AI safe in the workplace. Data security, hallucinations, biased outputs, and misuse (for example, creating harmful content or enabling fraud) are challenges that cannot be ignored. Employees are well aware of AI’s safety challenges. Their top concerns are cybersecurity, privacy, and accuracy (Exhibit 8). But what will it take for leaders to address these concerns while also moving ahead at light speed?
Image description begins:
The exhibit shows the share of US employees with concerns regarding gen AI, through a series of proportional area charts, each representing a specific risk associated with gen AI. The size of each chart indicates the percentage of US employees who cite that risk as a concern. Cybersecurity risks are cited by 51 percent of respondents, inaccuracies by 50 percent, and concerns about personal privacy by 43 percent. Intellectual property infringement is a concern for 40 percent of respondents, followed by workforce displacement (35 percent), explainability (34 percent), and equity and fairness (30 percent). Less prominent but still significant concerns were regulatory compliance issues (28 percent), national security (24 percent), damage to organizational reputation (16 percent), environmental impact (15 percent), physical safety (14 percent), and political stability (13 percent). Source: McKinsey US employee survey, Oct–Nov 2024. This image description was completed with the assistance of Writer, a gen AI tool.
Image description ends.
Employees trust business leaders to get it right
While employees acknowledge the risks and even the likelihood that AI may replace a considerable portion of their work, they place high trust in their own employers to deploy AI safely and ethically. Notably, 71 percent of employees trust their employers to act ethically as they develop AI. In fact, they trust their employers more than universities, large technology companies, and tech start-ups (Exhibit 9).
Image description begins:
The exhibit depicts the share of US employees who highly trust different institutions to deploy gen AI tools responsibly, safely, and ethically. The data is presented as four separate unit charts, each representing a distinct institution: employer, universities, large tech companies, and start-ups. Each unit chart consists of a 10x10 matrix of squares. The number of light blue squares within each grid represents the percentage of employees who express high trust in each institution. The remaining squares are light gray. Employers receive the highest level of trust (71 percent), followed by universities (67 percent), large tech companies (61 percent), and start-ups (51 percent). Source: McKinsey US employee survey, Oct–Nov 2024. This image description was completed with the assistance of Writer, a gen AI tool.
Image description ends.
According to our research, this is in line with a broader trend in which employees show higher trust in their employers to do the right thing in general (73 percent) than in other institutions, including the government (45 percent). This trust should help leaders act with confidence as they tackle the speed-versus-safety dilemma. That confidence also applies outside the United States, even though employees in other regions may have more desire for regulation (see sidebar “Global perspectives on regulation”).
Global perspectives on regulation
A high percentage of international C-suite leaders we surveyed across five regions (Australia, India, New Zealand, Singapore, and the United Kingdom) are Gloomers, who favor greater regulatory oversight. Between 37 to 50 percent of international C-suite leaders self-identify as Gloomers, versus 31 percent in the United States. This may be because top-down regulation is more accepted in many countries outside the United States. Of the global C-suite leaders surveyed, half or more worry that ethical use and data privacy issues are holding back their employees from adopting gen AI.
However, our research shows that attitudes about regulation are not inhibiting the economic expectations of business leaders outside the United States. More than half of the international executives (versus 41 percent of US executives) indicate they want their companies to be among the first adopters of AI, with those in India and Singapore being especially bullish (exhibit). The desire of international business leaders to be AI first movers can be explained by the revenue they expect from their AI deployments. Some 31 percent of international C-suite leaders say they expect AI to deliver a revenue uplift of more than 10 percent in the next three years, versus just 17 percent of US leaders. Indian executives are the most optimistic, with 55 percent expecting a revenue uplift of 10 percent or more over the next three years.
Risk management for gen AI
In Superagency, Hoffman argues that new risks naturally accompany new capabilities—meaning they should be managed but not necessarily eliminated.15 Leaders need to contend with external threats, such as infringement on intellectual property (IP), AI-enabled malware, and internal threats that arise from the AI adoption process. The first step in building fit-for-purpose risk management is to launch a comprehensive assessment to identify potential vulnerabilities in each of a company’s businesses. Leaders can then establish a robust governance structure, implement real-time monitoring and control mechanisms, and ensure continuous training and adherence to regulatory requirements.
One powerful control mechanism is respected third-party benchmarking that can increase AI safety and trust. Examples include Stanford CRFM’s Holistic Evaluation of Language Models (HELM) initiative—which offers comprehensive benchmarks to assess the fairness, accountability, transparency, and broader societal impact of a company’s AI systems—as well as MLCommons’s AILuminate tool kit on which researchers from Stanford collaborated.16 Other organizations such as the Data & Trust Alliance unite large companies to create cross-industry metadata standards that aim to bring more transparency to enterprise AI models.
While benchmarks have significant potential to build trust, our survey shows that only 39 percent of C-suite leaders use them to evaluate their AI systems. Furthermore, when leaders do use benchmarks, they opt to measure operational metrics (for example, scalability, reliability, robustness, and cost efficiency) and performance-related metrics (including accuracy, precision, F1 score, latency, and throughput). These benchmarking efforts tend to be less focused on ethical and compliance concerns: Only 17 percent of C-suite leaders who benchmark say it’s most important to measure fairness, bias, transparency, privacy, and regulatory issues (Exhibit 10).
Image description begins:
The exhibit presents data on the utilization of benchmarks for gen AI tools among US C-suite executives. The exhibit is in two parts. The first part is a pie chart showing that 39 percent of respondents have benchmark standards for gen AI tools used by their employees. This indicates a significant minority of C-suite executives currently employ such standards. The second part of the exhibit is a horizontal bar chart displaying the benchmarks considered most important by the C-suite respondents. Performance-related benchmarks are deemed most important by 41 percent of respondents. Operational benchmarks follow closely behind, cited by 35 percent of participants. Ethical and compliance benchmarks are a lower priority, selected by 17 percent of the respondents, while other benchmarks account for only 7 percent of responses. This reveals a noteworthy disparity, suggesting C-suite leaders put a stronger emphasis on benchmarking the performance and operational aspects of AI rather than benchmarking ethical considerations. Source: McKinsey US CxO survey, Oct-Nov 2024. This image description was completed with the assistance of Writer, a gen AI tool.
Image description ends.
The focus on operational and performance metrics reflects the understandable desire to prioritize immediate technical and business outcomes. But ignoring ethical considerations can come back to haunt leaders. When employees don’t trust AI systems, they are less likely to accept them. Although benchmarks are not a panacea to eliminate all risk and can’t ensure that AI systems are fully efficient, ethical, and safe, they are a useful tool.
Even companies that excel at all three categories of AI readiness—technology, employees, and safety—are not necessarily scaling or delivering the value expected. Nevertheless, leaders can harness the power of big ambitions to transform their companies with AI. The next chapter examines how.
Most organizations that have invested in AI are not getting the returns they had hoped. They are not winning the full economic potential of AI. About half of C-suite leaders at companies that have deployed AI describe their initiatives as still developing or expanding (Exhibit 11). They have had the time to move further. Our research shows that more than two-thirds of leaders launched their first gen AI use cases over a year ago.
This is a time when you should be getting benefits [from AI] and hope that your competitors are just playing around and experimenting.
Image description begins:
The exhibit is a horizontally stacked bar graph that shows the percentage of C-suite respondents who describe their gen AI rollouts by maturity stages. 8 percent of respondents report their organizations are in the nascent stage, characterized by minimal gen AI initiatives with no significant impact on employee workflows. A significantly larger portion, 39 percent, describe their organizations as being in the emerging stage, where gen AI pilot projects are starting to show value. The developing stage, where gen AI implementation is changing certain workflows and increasing efficiency, accounts for 31 percent of respondents. 22 percent of respondents place their organizations in the expanding stage, indicating that gen AI is scaled across departments, transforming workflows, and enhancing operations. Finally, only 1 percent of C-suite respondents describe their gen AI rollouts as mature, meaning that gen AI is fundamentally changing how work is done and driving substantial business outcomes. The exhibit highlights that the figures might not add up to 100 percent due to rounding. Source: McKinsey US CxO survey, Oct-Nov 2024. This image description was completed with the assistance of Writer, a gen AI tool.
Image description ends.
Pilots fail to scale for many reasons. Common culprits are poorly designed or executed strategies, but a lack of bold ambitions can be just as crippling. This chapter looks at patterns governing today’s investments in AI across industries and suggests the potential awaiting those who can dream bigger.
AI investments vary by industry
Different industries have different AI investment patterns. Within the top 25 percent of spenders, companies in healthcare, technology, media and telecom, advanced industries, and agriculture are ahead of the pack (Exhibit 12). Companies in financial services, energy and materials, consumer goods and retail, hardware engineering and construction, and travel, transport, and logistics are spending less. The consumer industry—despite boasting the second-highest potential for value realization from AI—seems least willing to invest, with only 7 percent of respondents qualifying in the top quartile, based on self-reported percentage of revenue spend on gen AI. That hesitation may be explained by the industry’s low average net margins in mass-market categories and thus higher confidence thresholds for adopting costly organization-wide technology upgrades.
Image description begins:
The scatterplot exhibit depicts how companies’ gen AI spend does not match the economic potential in their industries. The exhibit illustrates that several industries with a high economic potential from gen AI are not yet spending significantly on the technology. It shows the relationship between the industry share of overall survey respondents and the industry share of top-quartile gen AI spending. On both axes, the top value is set to 35 percent. The size of each circle represents the economic potential from gen AI in billions of dollars for each industry.
Light blue circles represent industries where the share in the top quartile of gen AI spending is higher than their overall survey share. These include healthcare, which has a large circle indicating significant economic potential from gen AI, and technology, also with a substantial circle suggesting large economic potential. Media and telecom and advanced Industries are also shown in light blue to illustrate strong economic potential from gen AI, but with smaller circles indicating less economic potential than healthcare and technology. Agriculture is represented by a small light blue circle.
Dark grey circles represent industries where the share in the top quartile of gen AI spending is lower than their overall survey share. These include financial services, which has a large circle showing high economic potential. Energy and materials, consumer goods and retail, hardware engineering and construction, and travel, transportation, and logistics are represented by overlapping circles of varying sizes, implying a range of economic potential.
No industry scores higher than 20 percent on the share of overall survey respondents. Some industries such as media and telecom, advanced Industries, and agriculture account for around 5 percent or less of overall survey respondents. Industries that scored high percentages on the share of top-quartile gen AI spending include healthcare and technology.
Source: The economic potential of gen AI: The next productivity frontier, McKinsey. This image description was completed with the assistance of Writer, a gen AI tool.
Image description ends.
In some industries, employees are cautious
Employees in the public sector, as well as the aerospace and defense and semiconductor industries, are largely skeptical about the development of AI’s future. In the public sector and aerospace and defense, only 20 percent of employees anticipate that AI will have a significant impact on their daily tasks in the next year, versus roughly two-thirds in media and entertainment (65 percent) and telecom, at 67 percent (Exhibit 13). What’s more, our survey shows that just 31 percent of social sector employees trust that their employers will develop AI safely. That’s the least confidence in any industry; the cross-industry average is 71 percent.
Employees’ relative caution about AI in these sectors likely reflects near-term challenges posed by external constraints such as rigorous regulatory oversight, outdated IT systems, and lengthy approval processes.
There’s a lot of headroom in some functions
Our research finds that the functional areas where AI presents the greatest economic potential are also those where employee outlook is lukewarm. Employees in sales and marketing, software engineering, customer service, and R&D contribute roughly three-quarters of AI’s total economic potential, but the self-reported optimism of employees in these functions is middling (Exhibit 14). It may be the case that these functions have piloted AI projects, leading employees to be more realistic about AI’s benefits and limitations. Or perhaps the economic potential has made them worry that AI could replace their jobs. Whatever the reasons, leaders in these functions might consider investing more in employee support and elevating the change champions who can improve that sentiment.
Image description begins:
The exhibit is made up of a scatter plot and a separate bar chart visualizing the relationship between the potential economic value from gen AI and the share of employees with a positive outlook on gen AI, categorized by business function. The exhibit illustrates that the functions with the employees most optimistic about gen AI are not the functions with the greatest potential economic value from gen AI.
The scatter plot displays business functions: sales and marketing, software engineering, customer service, R&D, legal, risk, and compliance, operations, HR, strategy, supply chain, finance, procurement, and IT. Each function is represented by a data point, with its horizontal position indicating the percentage of employees expressing a positive outlook on gen AI, and its vertical position representing the potential economic value of gen AI in those functions, in trillions of dollars. Sales and marketing shows the highest potential economic value and around 50 percent of employees with a positive outlook. Software engineering is the function with second-highest economic potential from gen AI, with again about 50 percent of employees in that function reporting being optimistic about gen AI. Customer service and R&D also show about 50 percent of employees with a positive outlook on gen AI, but a much lower potential economic value. Several functions, such as operations, HR, Strategy, and IT, cluster together with low potential economic value and similarly middling employee optimism. Employes in IT, finance, and procurement are the most optimistic about gen AI, with about 70 percent of employees reporting positive sentiment, but these functions represent low economic potential from gen AI.
The adjacent bar chart breaks down the share of the total potential economic value contributed by each function. Sales and marketing accounts for 28 percent of the total potential economic value from gen AI, followed by software engineering at 25 percent. Customer service contributes 11 percent, while R&D contributes 9 percent. The remaining 27 percent is attributed to other functions. Source: McKinsey US CxO survey, Oct-Nov 2024. This image description was completed with the assistance of Writer, a gen AI tool.
Image description ends.
Gen AI has not delivered enterprise-wide ROI, but that can change
Across all industries, surveyed C-level executives report limited returns on enterprise-wide AI investments. Only 19 percent say revenues have increased more than 5 percent, with another 39 percent seeing a moderate increase of 1 to 5 percent, and 36 percent reporting no change (Exhibit 15). And only 23 percent see AI delivering any favorable change in costs.
Image description begins:
In this exhibit, a pair of segmented bar charts displays US CxOs’ perceptions of whether gen AI has delivered significant return on investment for their enterprises, with details on whether they believe gen AI has impacted their revenues and costs. The data is divided into two bar graphs, one for revenues and one for costs, each presented as a stacked bar chart showing the percentage of respondents who report various levels of change.
In the revenues section, 39 percent of respondents report that gen AI has delivered a revenue increase of 1–5 percent, 12 percent report an increase of 6–10 percent, and 7 percent report a revenue increase of more than 10 percent. A significant 36 percent report no change in revenue, while a small percentage (2 percent) report a decrease. An additional 3 percent are not tracking revenue related to gen AI, and 2 percent indicate they do not know.
The costs section presents a similar breakdown. A substantial 31 percent of respondents report that gen AI has resulted in no change in their organizations’ costs, followed by 29 percent who report an increase of 1–10 percent. Furthermore, 17 percent report a cost decrease of 1–10 percent, while 6 percent report a decrease of 11–19 percent. A smaller percentage of 10 percent indicate a cost increase of 11–19 percent, while 4 percent report a cost increase of 20 percent or more. Similar to the revenue section, 2 percent of respondents are not tracking cost changes related to gen AI, and 3 percent indicate they do not know. The exhibit highlights that the figures might not add up to 100 percent due to rounding. Source: McKinsey US CxO survey, Oct-Nov 2024. This image description was completed with the assistance of Writer, a gen AI tool.
Image description ends.
Despite this, company leaders are optimistic about the value they can capture in the coming years. A full 87 percent of executives expect revenue growth from gen AI within the next three years, and about half say it could boost revenues by more than 5 percent in that time frame (Exhibit 16). That suggests quite a lot could change for the better over the next few years.
Image description begins:
In this exhibit, a segmented bar graph shows the extent to which C-suite executives perceive gen AI will affect their organizations’ revenues over the next three years, and the percentage of respondents who anticipate gen AI will result in different levels of revenue change. The bar graph shows that 36 percent of respondents anticipate that gen AI will deliver a 1-5 percent increase in revenue, 34 percent anticipate a 6-10 percent increase in revenue, and 17 percent anticipate a greater than 10 percent increase in revenue. In contrast, 10 percent of respondents anticipate that gen AI will deliver no change in revenue. A total of 51 percent of respondents anticipate that gen AI will deliver a revenue increase of over 5 percent. No respondents anticipate that gen AI will deliver a decrease in revenue, while 3 percent are not currently tracking revenue changes related to gen AI. Source: McKinsey US CxO survey, Oct-Nov 2024. This image description was completed with the assistance of Writer, a gen AI tool.
Image description ends.
Big ambitions can help solve big problems
To drive revenue growth and improve ROI, business leaders may need to commit to transformative AI possibilities. As the hype around AI subsides and the focus shifts to value, there is a heightened attention on practical applications that can create competitive moats.
[It] is critical to have a genuinely inspiring vision of the future [with AI] and not just a plan to fight fires.
To assess how far along companies are in this shift, we examined three categories of AI applications: personal use, business use, and societal use (see sidebar “AI’s potential to enhance our personal lives”). We mapped over 250 applications from our work and publicly shared examples to understand the spectrum of impact levels, from localized use cases to transformations with more universal impact. Our conclusion? Given that most companies are early in their AI journeys, most AI applications are localized use cases still in the pilot stages (Exhibit 17).
Image description begins:
This text-based exhibit illustrates how gen AI use cases can be categorized by their impact levels, ranging from localized impact to universal impact. The text table presents three sections of gen AI deployments: use cases, domains, and transformations. Within each section, examples of gen AI applications are shown, with colored dots indicating whether the type of primary impact for each example is personal, business, or societal. The use cases section focuses on gen AI deployments that provide productivity boosts through automation of specific tasks or jobs. Examples include conducting smarter searches for everyday information (personal), planning events (personal), assessing candidate recruiting performance (business), accelerating contract generation (business), processing customer information faster (business), and identifying high-value consumers for tailored sales actions (business). These gen AI examples are all positioned on the more localized end of the impact spectrum.
The domains section shows gen AI applications that reshape multiple roles across an area of operations. These are all classified as having a primary business impact and include developing and executing data-based campaigns, conducting synthetic customer research, conducting real-time supply chain monitoring, and accelerating coding processes. These use cases fall in the middle of the spectrum between more localized and more universal impact levels.
Finally, the transformations section highlights use cases that fundamentally reshape industries, fields, and lives. These are positioned at the more universal end of the impact spectrum and include accelerating discovery and manufacturing in material science (business), predicting natural disasters and supporting crisis management strategy (societal), and accelerating drug development by reducing cost and time (societal). This image description was completed with the assistance of Writer, a gen AI tool.
Image description ends.
In many cases, that’s perfectly appropriate. But creating AI applications that can revolutionize industries and create transformative value requires something more. Robotics in manufacturing, predictive AI in renewable energy, drug development in life sciences, and personalized AI tutors in education—these are the kinds of transformative efforts that can drive the greatest returns.17 These weren’t created from a reactive mindset. They are the result of inspirational leadership, a unique concept of the future, and a commitment to transformational impact. This is the kind of courage needed to develop AI applications that can revolutionize industries.
It is in [the] collaboration between people and algorithms that incredible scientific progress lies over the next few decades.
To truly harness the potential of AI, companies must challenge themselves to envision and implement more breakthrough initiatives. Success in the era of AI hinges not just on technology deployment or employee willingness but also on visionary leadership. The ingredients are here. The technology is already highly capable and rapidly advancing, and employees are more ready than leaders think. Leaders have more permission space than they realize to deploy AI quickly in the workplace. To do so, leaders need to stretch their ambitions toward systematic change, laying the foundation for real competitive differentiation. If they want to be more ambitious about AI, companies must increase the proportion of transformational initiatives in their portfolios. The next chapter examines the headwinds that leaders must overcome—and how they can do so.
AI’s potential to enhance our personal lives
Outside of the business context, individuals are increasingly using AI in their personal lives. In previous research, we analyzed the potential impact of AI across 77 personal activities and across age, gender, and working status in the United States. While individuals have limited desire to automate certain personal activities, including leisure, sleeping, and fitness, the data shows significant opportunity for AI combined with other technologies to help with chores or labor-intensive tasks. Already in 2024, our research identified about an hour of such daily activities with the technical potential to be automated. By 2030, expansion of use cases and continued improvements in AI safety could increase automation potential up to three hours per day. When people use AI-enabled tools—say, an autonomous vehicle for transportation or an interactive personal finance bot—they can repurpose time for personal fulfillment activities or being productive in other ways.
Using human-centric design and tapping into gen AI’s potential for “emotional intelligence” are unlocking new personal AI applications that go beyond basic efficiencies. Individuals are beginning to use conversational and reasoning AI models for counseling, coaching, and creative expression. For example, people are using conversational AI for advice and emotional support or to bring their artistic visions to life with only verbal cues. Further, to the notion that AI superagency will advance society, AI has potential to become a democratizing force, making experiences that were previously expensive or exclusive—such as animation generation, career coaching, or tax advice—available to much wider audiences.
There is no question: AI offers a rare and phenomenal opportunity. Almost 90 percent of leaders anticipate that deploying AI will drive revenue growth in the next three years. But securing that growth entails corporate transformation, and businesses have a poor track record in this area. Nearly 70 percent of transformations fail.
As we build this next generation of AI, we made a conscious design choice to put human agency both at a premium and at the center of the product. For the first time, we have the access to AI that is as empowering as it is powerful.
To make their companies part of the minority that succeed, C-level executives must turn the mirror on themselves. They need to embrace the vital role their leadership plays. C-suite leaders participating in our survey are more than twice as likely to say employee readiness is a barrier to adoption as they are to blame their own role. But as previously noted, employees indicate that they are quite ready.
This chapter looks at how leaders can take the reins, recognizing and owning the fact that the AI opportunity requires more than technology implementation. It demands a strategic transformation. There is no denying that companies face a set of AI headwinds. To tackle these challenges, leadership teams will need to commit to rewiring their enterprises.
The operational headwinds that slow execution
Business adoption of AI faces several operational headwinds. Our interviews and research surfaced five that are most challenging: aligning leadership, addressing cost uncertainty, workforce planning, managing supply chain dependencies, and meeting the demand for explainability.
Leadership alignment is a challenging but critical first step
Securing consensus from senior leaders on a strategy-led gen AI road map is no simple task. The key to meeting this challenge is first recognizing that leadership alignment cannot be oversimplified or assumed. The process requires ongoing engagement from senior leaders across business domains, each of which may have distinct objectives and risk appetites. Together, leaders must clearly define where value lies, how AI will drive this value, and how risk will be mitigated. They must collectively establish metrics for performance evaluation and investment recalibration. To facilitate alignment, they may want to appoint a gen AI value and risk leader or institute an enterprise-wide leadership and orchestration function. These actions can enhance collaboration among business, technology, and risk teams. Although challenging, aligning leadership is a crucial step to ensure that AI projects are not disparate, avoid liability, and deliver transformative business outcomes.
Cost uncertainty makes it difficult for enterprises to predict ROI
Many companies are still determining if they can “take” AI solutions off the shelf from tech vendors or if they need to “shape” and customize them, which can be more costly but brings the potential for greater differentiation from competitors. Additionally, while leaders can budget for AI pilots, the full cost of building and managing AI applications at scale remains uncertain. Planning for a limited pilot is very different from assessing the costs of a mature solution that helps most employees multiple times a day. These factors lead to tough tradeoffs. But to move at the pace of AI, technology leaders must prioritize accelerated decision-making.
Workforce planning is more difficult than ever
There is still a world of uncertainty to manage. Employers do not know how many AI experts they will need with what type of skills, whether that talent bench even exists, how quickly they can source people, and how they can remain an attractive employer for in-demand hires after they come aboard. On the other hand, they do not know how fast AI may depress demand for other skills and thus require workforce rebalancing and retraining.
Supply chain dependencies can wreak havoc
Fragile supply chains can expose enterprises to disruptions and technical, regulatory, and legal challenges. The AI supply chain is global, with significant R&D concentrated in China, Europe, and North America and with semiconductor and hardware manufacturing concentrated in East Asia and the United States. Today’s geopolitics are complex. Furthermore, models and applications are increasingly created in open-source forums spanning many countries.
Demand for greater explainability is a central challenge
Safe AI deployment is increasingly a must-have. Yet most LLMs are often black boxes that do not reveal why or how they came to a certain response, nor what data was used to make it. If AI models cannot provide clear justifications for their responses, recommendations, decisions, or actions—showing the specific factors that led to a credit card application denial, for example—they will not be trusted for critical tasks.
These AI-specific headwinds are formidable but addressable. Companies are pushing ahead. For example, they might use dynamic cost planning or look at procuring NVIDIA clusters to secure the infrastructure they expect to need.18 Chief HR officers (CHROs) are developing training programs to upskill their current workforces and support some employees in job transitions. But lasting success will take more than that.
To capture AI value, leaders must rewire their companies
McKinsey’s Rewired framework includes six foundational elements to guide sustained digital transformation: road map, talent, operating model, technology, data, and scaling (Exhibit 18). When companies implement this playbook successfully, they cultivate a culture of autonomy, leverage modern cloud practices, and assemble multidisciplinary agile teams.
Image description begins:
The text-based exhibit is a framework outlining six key success factors for tech-business transformations. It's structured as a grid with a title, “Framework for the coordinated execution of value creation," and is further divided into six rectangular sections, each representing a different success factor. An overarching section, business-led digital road map, focuses on the importance of an organization aligning senior leadership to create a clear vision, value proposition, and roadmap for transformation, thereby improving the customer experience and competitiveness. The next four sections are categories representing the core operational aspects of a business-tech transformation. Talent emphasizes the importance of having employees with the right skills and capabilities to execute and innovate. Operating model highlights the need for organizations to relentlessly focus on value creation by integrating business, technology, and operations. Technology stresses the importance of using technology effectively through adoption of the right platforms, solutions, and practices to drive innovation. Finally, data and AI focuses on why it’s essential to provide people in the organization with easy access to high-quality data and to leverage AI insights to enhance customer experiences and business operations. The final section, underpinning the four category sections, is activation and scaling. This section underscores the need for organizations to maximize value capture by ensuring the activation and enterprise scaling of digital solutions, while carefully managing the transformation's progress and mitigating risks. This image description was completed with the assistance of Writer, a gen AI tool.
Image description ends.
While these six elements are universally applicable, AI has introduced a few important wrinkles for leaders to address:
- Adaptability. AI technology is advancing so rapidly that organizations must adopt new best practices quickly to stay ahead of the competition. Best practices may come in the form of new technologies, talent, business models, or products. For example, a modular approach helps future-proof tech stacks. As natural language becomes a medium for integration, AI systems are becoming more compatible, allowing businesses to swap, upgrade, and integrate models and tools with less friction. This modularity allows enterprises to avoid vendor lock-in and put new AI advancements to use quickly without constantly reinventing their tech stacks.
- Federated governance models. Managing data and models can give teams autonomy to develop new AI tools while centrally controlling risk. Leaders can directly oversee high-risk or high-visibility issues, such as setting policies and processes to monitor models and outputs for fairness, safety, and explainability. But they can set direction and delegate other monitoring to business units, including measuring performance-based criteria such as accuracy, speed, and scalability.
- Budget agility. Given technological advances across models, as well as the opportunity to curate an optimal mix of LLMs, small language models (SLMs), and agents, business leaders should keep their budgets flexible. This helps enterprises optimize their AI deployments simultaneously for costs and performance.
- AI benchmarks. These tools can serve as powerful means to quantitatively assess, compare, and improve the performance of different AI models, algorithms, and systems. If technologists come together to adopt standardized public benchmarks—and if more C-level executives start employing benchmarks, including ethical ones—model transparency and accountability will improve and AI adoption will increase, even among more skeptical employees.
- AI-specific skill gaps. Notably, 46 percent of leaders identify skill gaps in their workforces as a significant barrier to AI adoption. Leaders will need to attract and hire top-level talent, including AI/ML engineers, data scientists, and AI integration specialists. They will also need to commit to creating an environment that is attractive to technologists. For example, this can mean providing them with plenty of time to experiment, offering access to cutting-edge tools, creating opportunities to engage in open-source communities, and promoting a collaborative engineering culture. Upskilling existing employees is just as critical: Research from McKinsey’s People and Organizational Performance Practice underscores the importance of tailoring training to specific roles, such as offering technical team members bootcamps on library creation while offering prompt engineering classes to specific functional teams.19
- Human centricity. To guarantee both fairness and impartiality, it is important that business leaders incorporate diverse perspectives early and often in the AI development process and maintain transparent communication with their teams. As it stands, less than half of C-suite leaders (48 percent) say they would involve nontechnical employees in the early development stages of AI tools, specifically ideation and requirement gathering. Agile pods and human-centric development practices such as design-thinking and reinforcement learning from human feedback (RLHF) will help leaders and developers create AI solutions that all people want to use. In agile pods, technical team members sit alongside employees from business functions such as HR, sales, and product, and from support functions such as legal and compliance. Further, leaders can empathize with employees’ uneasiness about AI’s impacts on potential job losses by being honest about new skill requirements and head count changes. Forums where employees can provide input on AI applications, voice concerns, and share ideas are valuable for maintaining a transparent, human-first culture.
The pace at which AI has advanced over the last two years is stunning. Some react to that pace by seeing AI as a challenge to humanity. But what if we take the advice of Reid Hoffman and imagine what could possibly go right with AI? Leaders might realize that all the pieces are in place for AI superagency in the workplace.
Learn from yesterday, live for today, hope for tomorrow.
They might notice that their employees are already using AI and want to use it even more. They may find that millennial managers are powerful change champions ready to encourage their peers. Instead of focusing on the 92 million jobs expected to be displaced by 2030, leaders could plan for the projected 170 million new ones and the new skills those will require.20
This is the moment for leaders to set bold AI commitments and to meet employee needs with on-the-job training and human-centric development. As leaders and employees work together to reimagine their businesses from the bottom up, AI can evolve from a productivity enhancer into a transformative superpower—an effective partner that increases human agency. Leaders who can replace fear of uncertainty with imagination of possibility will discover new applications for AI, not only as a tool to optimize existing workflows but also as a catalyst to solve bigger business and human challenges. Early stages of AI experimentation focused on proving technical feasibility through narrow use cases, such as automating routine tasks. Now the horizon has shifted: AI is poised to unlock unprecedented innovation and drive systemic change that delivers real value.
To meet this more ambitious era, leaders and employees must ask themselves big questions. How should leaders define their strategic priorities and steer their companies effectively amid disruption? How can employees ensure they are ready for the AI transition coming to their workplaces? Questions like the following ones will shape a company’s AI future:
For business leaders:
- Is your strategy ambitious enough? Do you want to transform your whole business? How can you reimagine traditional cost centers as value-driven functions? How do you gain a competitive advantage by investing in AI?
- What does successful AI adoption look like for your organization? What success indicators will you use to evaluate whether your investments are yielding desired ROI?
- What skills define an AI-native workforce? How can you create opportunities for employees to develop these skills on the job?
For employees:
- What does achieving AI mastery mean for you? Does it extend to confidently using AI for personal productivity tasks such as research, planning, and brainstorming?
- How do you plan to expand your understanding of AI? Which news sources, podcasts, and video channels can you follow to remain informed about the rapid evolution of AI?
- How can you rethink your own work? Some of the most innovative ideas often emerge from within teams, rather than being handed down from leadership. How would you redesign your work to drive bottom-up innovation?
These questions have no easy answers, but a consensus is emerging on how to best address them. For example, some companies deploy both bottom-up and top-down approaches to drive AI adoption. Bottom-up actions help employees experiment with AI tools through initiatives such as hackathons and learning sessions. Top-down techniques bring executives together to radically rethink how AI could improve major processes such as fraud management, customer experience, and product testing.
These kinds of actions are critical as companies seek to move from AI pilots to AI maturity. Today only 1 percent of business leaders report that their companies have reached maturity. Over the next three years, as investments in the technology grow, leaders must drive that percentage way up. They should make the most of their employees’ readiness to increase the pace of AI implementation while ensuring trust, safety, and transparency. The goal is simple: capture the enormous potential of gen AI to drive innovation and create real business value.